Risks of Synthetic Data Teaching
2 min read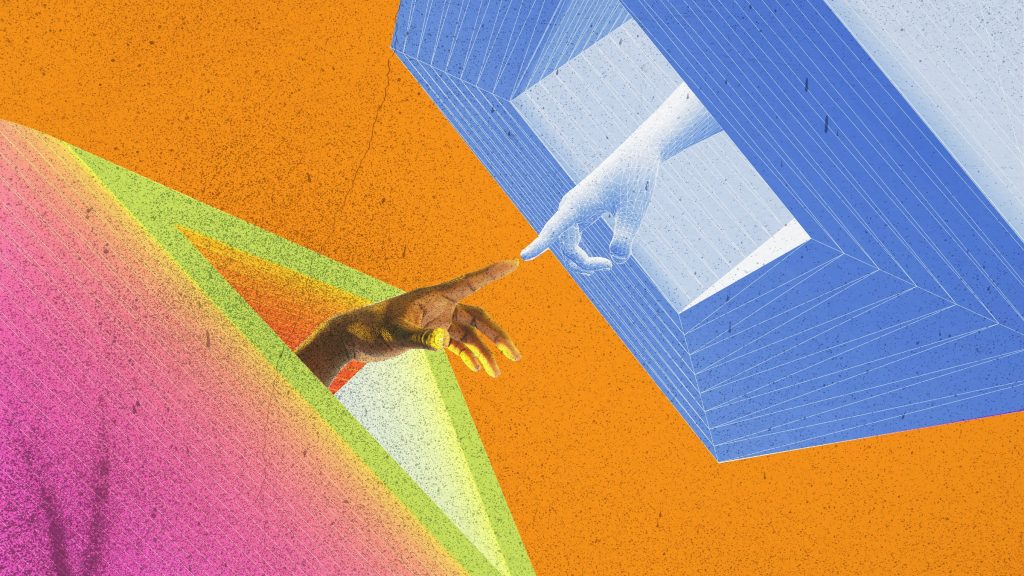
Synthetic Data Is a Dangerous Teacher
Synthetic data, also known as artificial data or simulated data, is data that is artificially created rather than being generated by actual events. While synthetic data can be useful in certain applications, it also comes with risks and limitations, particularly when used as a teaching tool.
One of the dangers of synthetic data is that it may not accurately reflect the complexities and nuances of real-world data. This can lead to biased or misleading results when using synthetic data to train machine learning models or analyze trends. For example, synthetic data may not capture the full range of variations and outliers present in real data, leading to models that perform poorly when applied to real-world scenarios.
Furthermore, synthetic data can also perpetuate existing biases and stereotypes present in the data used to create it. If the synthetic data is based on biased or incomplete real data, the resulting synthetic data will also be biased and may reinforce harmful stereotypes or discriminatory practices.
While synthetic data can be a useful tool in certain contexts, it is important to approach its use with caution and skepticism. It is essential to validate and test synthetic data thoroughly to ensure that it accurately represents the real-world phenomena it is meant to model. Additionally, it is crucial to consider the ethical implications of using synthetic data, particularly in sensitive or high-stakes applications.
In conclusion, synthetic data can be a dangerous teacher if used carelessly or uncritically. It is important to approach synthetic data with caution, skepticism, and a critical eye to ensure that it is used responsibly and ethically.